Generalized Linear Models With Examples in R
ebook ∣ Springer Texts in Statistics
By Peter K. Dunn
Sign up to save your library
With an OverDrive account, you can save your favorite libraries for at-a-glance information about availability. Find out more about OverDrive accounts.
Find this title in Libby, the library reading app by OverDrive.
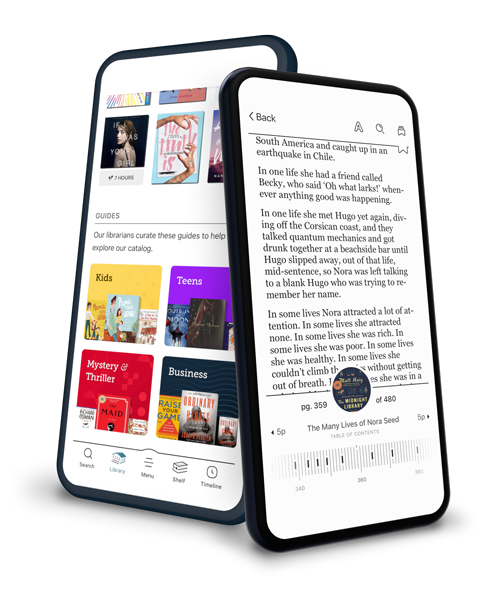
Search for a digital library with this title
Title found at these libraries:
Loading... |
This textbook presents an introduction to generalized linear models, complete with real-world data sets and practice problems, making it applicable for both beginning and advanced students of applied statistics. Generalized linear models (GLMs) are powerful tools in applied statistics that extend the ideas of multiple linear regression and analysis of variance to include response variables that are not normally distributed. As such, GLMs can model a wide variety of data types including counts, proportions, and binary outcomes or positive quantities.
Other features include: